Edge AI use cases in industries are wide ranging, from quality control in manufacturing lines to safety monitoring of human-machine interaction. These applications need fast, low latency inference without compromising on accuracy.
20% of leading manufacturers will rely on embedded intelligence by 2021. AI, IoT and blockchain applications are expected to increase execution times by up to 25%.
Source: IDC
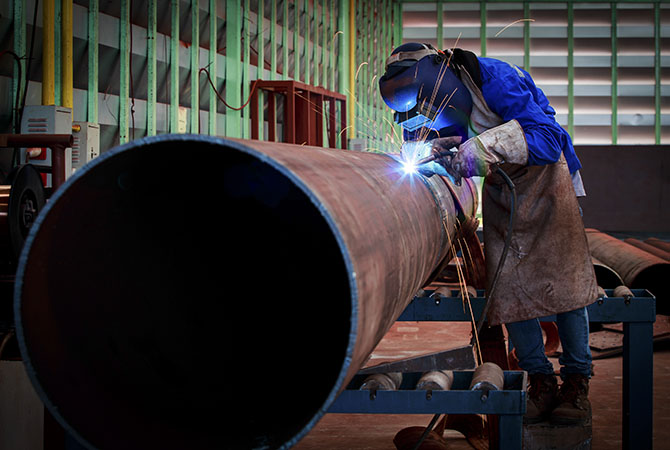